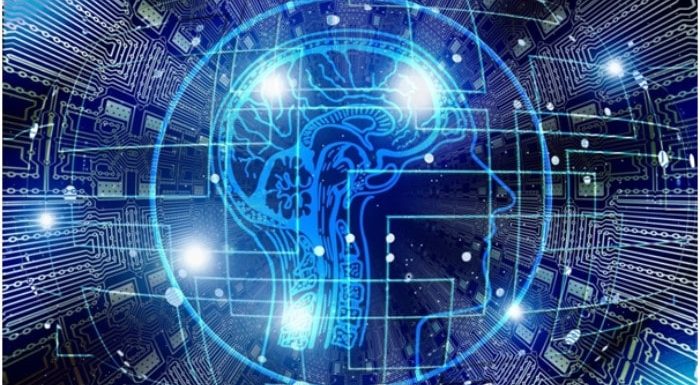
If you’ve been around the tech community lately, you’ve probably heard these acronyms—AI, ML, Deep Learning, and Data Science—being tossed around by investors, entrepreneurs, and innovators alike.
Moreover, the world of data science and machine learning (ML) can be overwhelming, especially if you’re new to the field. It seems like there are new algorithms and terms popping up every day, and it’s hard to keep track of them all!
To make matters worse, you may have noticed the trend towards Artificial Intelligence (AI), Machine Learning (ML), and Deep Learning (DL) in business lately; some companies even claim to have ML algorithms that can replace human employees entirely!
This makes it increasingly critical to understand the differences between these terms and how they each fit into modern business models and corporate strategies.
If you’re like other people in the tech world, though, you probably have no clue about what these technologies mean or how they differ from one another. This article will explain each of these terms and more so that you can start using them with confidence!
Artificial intelligence:
AI is a vast category with many different subsets. Deep learning is one type of AI that makes it possible for machines to learn tasks on their own without being specifically programmed to perform them.
Something that’s obviously very appealing to businesses looking for ways to cut costs while maximizing productivity.
The term artificial intelligence has also been used more generally to refer to smart or intelligent computer systems or software in general, even when they aren’t deep learning applications.
In fact, AI itself isn’t a field but rather an umbrella term covering many specific areas of machine learning research and development. AI innovation is becoming a sole practice at AI development companies, and they are trying to make every area more advanced with it.
This can be a bit chaotic, so let’s simplify things: Artificial intelligence (AI) is made up of three major subfields: Narrow AI, General AI, and Strong AI.
Narrow AI refers to situations where computers are trained for highly specific tasks.
In contrast, General AI describes computer systems capable of performing complex human cognitive functions such as decision making.
Strong AI is AI that is more intelligent than humans and can be more advanced & threatening.
However, AI is simply an umbrella term that includes practices of machine learning, deep learning, natural language processing, and much more.
Today, every AI development company is engaged in harnessing the potential of this technology in every way possible to provide humans with a world of ease & leisure.
Machine Learning:
ML is a subset of AI that focuses on getting computers to learn without being explicitly programmed.
Machine learning allows the software to automatically identify patterns in data—such as images, text, or sounds—without being explicitly programmed where these patterns are.
ML software uses statistics to get more accurate over time. ML is commonly used for applications like speech recognition, email spam filtering, facial recognition (Robo-signing), autonomous vehicles…and more!
It’s also an essential part of many other subfields within AI: natural language processing (NLP), computer vision (CV), etc. This leads us to another related area: deep learning.
Deep Learning:
AI’s cousin, machine learning, is often confused with artificial intelligence. These terms are not synonyms. Machine learning is a component of AI (it focuses on getting computers to solve problems autonomously), but it is not an end goal.
Deep learning refers to a subset of machine learning where artificial neural networks (ANNs) are used to learn representations of data that can be used for prediction or classification, essentially teaching machines how to see and hear as humans do.
One of deep learning’s biggest advantages is its capacity to teach computers to learn with minimal guidance.
Instead of explicitly programming every little decision that a machine might need to make, you can give it a large amount of data and then let it figure out how to extract information on its own.
This has huge implications for business—after all if you could teach your computer all of your organization’s processes without writing down every single step, imagine how much time you could save.
Data Science
The idea of data science has become a buzzword—if not an outright fad—that is often used to describe statistics or other quantitative approaches to solving business problems.
However, there is a difference between data science and statistical analysis. Although they share some similarities (particularly in math), data science is more focused on end-user understanding.
With data science tools like machine learning algorithms and predictive models, computers can not only compile statistics but also help people make sense of it all.
Moreover, AI development companies also help in leveraging the true potential of Data science practices with smart software.
In short, data science is concerned with making predictions based on existing data to develop better models for future use.
Comparison Between AI Vs ML Vs Deep Learning VS Data Science
-
AI VS ML Vs Deep Learning Vs Data Science Applications
Applications of AI technology:
- Self-driving cars (computers that drive without human input)
- Recommendation engines ( For reference, those used by Amazon to suggest products or Netflix recommend movies)
- Face recognition software Predictive analytics (software that can predict future outcomes based on past experiences)
Applications OF Machine Learning:
- The possibilities for ML are nearly endless: oncology, space exploration,
- Image/video recognition (think robots that recognize your face as you enter a room)
- Fraud detection, big data analysis…the list goes on!
Applications Of Deep Learning :
- Speech Recognition & Machine Translation,
- Sentiment Analysis & Recommendation Engines,
- Computer Vision for Image Classification, Object Detection, &Human Pose Estimation
- Fraud Detection, Breast Cancer Classification, Wine Classification, and more.
It’s important to note that many areas of AI fall under different umbrella terms.
Succinctly, it’s up to you which term works for you- ML, DL, or AI. For more clarity, you can rely on an AI & machine learning development company to bring your idea into digital reality.
Application Of Data Science:
- Fraud and Risk Detection
- Medical Image Analysis
- Targeted Advertising
- Advanced Image Recognition
- Finding biological connections between genetics, diseases, and drug response.
- Virtual assistance for patients and customer support
- Advanced Image Recognition
A Quick Look Into Machine Learning Vs Deep Learning:
-
AI Vs ML Vs Deep Learning Data Science: How They Can Help Us?
Artificial Intelligence:
AI can do some pretty amazing things! Today’s fastest computers are already capable of solving problems that would have been considered science fiction only a few years ago.
- AI tools can learn on their own through massive amounts of data. They can also process much faster than humans (sometimes thousands of times faster).
- This combination of a machine learns on its own through exposure to large data sets and is able to think more quickly than humans.
- This gives businesses access to capabilities like predictive analytics, self-driving cars, speech recognition software, and more.
- Robots can talk like humans via a Speech recognition system. Chatbots and conversational AI are some examples of that.
Some people think AI will eventually replace humans altogether; others believe it will only serve to make us smarter and more efficient in our work.
Now we’re working to develop even more sophisticated computer systems capable of handling complex scenarios like language translation, image analysis, text generation, pattern detection, and so much more.
If you are striving to leverage AI’s perk within your business, you can hire AI developers with innovative approaches & expertise. It is critical in 2022 to think with a broader objective and tech perspective.
Machine Learning:
For business, machine learning has several practical applications.
- If your company has a large customer database, you can use ML to identify potential customers more efficiently by analyzing which specific traits most frequently lead to successful sales.
- It can aid you in saving your business a lot of time (and money) spent on new advertising campaigns or other initiatives that don’t get results!
Alternatively, many companies today rely heavily on data collected from user interactions with their products; they might track things like how much time users spend with various pages on their site or how far they scroll down an email newsletter.
If you are pondering about creating a smart system for your business that can drive more customers and revenue, It is critical that you hire machine learning developers with a critical innovation approach to meet exact business needs.
Deep Learning:
Deep Learning can provide better solutions to classification problems.
- It can recognize patterns that are too complex for other machine learning algorithms. It can scale much better than traditional machine learning solutions.
- It is computationally expensive. Developing ANNs is still a long process (it takes months). You will also need extremely high-quality data to get good results;
- Otherwise, your accuracy will be severely compromised—and you’ll have no idea why your model works when deployed on real data but fails during testing.
And don’t even think about applying deep learning to products with an excessively large number of variables—unless you have limitless resources at your disposal!
Facebook uses deep learning algorithms to classify faces in photos. Skype uses it in their live translation product.
When you hire machine learning developers, they can provide you with deep learning solutions as well. You just need to put your idea clearly to secure a solution specific to your business.
Data Science:
The Scope Of Data Science is broader than AI, ML, and Deep Learning.
- The deeper level of inquiry requires a truly multidisciplinary approach that combines technical skills and real-world experience across various industries.
- It’s not just about crunching numbers anymore. Businesses can use data science to understand who customers are and what they need, driving decisions through evidence rather than instinct alone.
- It can help in finding answers to the questions such as: how will customers respond to pricing changes? Which new product features will drive engagement? How do we get users started as quickly as possible? And so on.
But building solutions at scale involves more than just developing technologies and putting them into production.
A mature data science team should include full-stack engineers, modelers, data architects, and visualization experts—people who will interact with stakeholders throughout an organization to solve key challenges facing your company today.
Some businesses hire machine learning developers to create business-specific data representation systems that can process large data and cater to more informed insights.
Succinctly, applications of data science are huge that can help businesses streamline different channels of revenue.
-
AI Vs Deep Learning Vs Machine Learning: Which Industries Can Use?
Industries That Can Use AI:
- Transportation
- Healthcare
- Finance
- Manufacturing Industries
- Automobile
- Advertising, and more
Industries That Can Use Machine Learning :
- Ceramics
- Automotive
- Energy management
- Food and beverage markets
- Ecommerce & Retail
- Entertainment
Industries That Can Use Deep Learning
- Digital Assistance
- Energy
- Hospitality
- Manufacturing
- Cybersecurity
- Agriculture and more.
Industries That Can Use Data Science:
- BFS
- Media & Entertainment.
- Healthcare
- Retail
- Telecommunications
- Automotive
- Digital Marketing.
- Professional Services
Apart from their industry application, whether you hire AI developers or data scientists, every one of them has a different role to perform. Only you have to decide objectives and look for the term that works for you the best.
-
AI Vs ML Vs Deep Learning Vs Data Science: Final Verdict
Wrapping Up
While data science is all about statistics, data, and making predictions through processing all gathered information, ML, AI, and deep learning are completely different from it.
Precisely, all of these technologies are connected to each other and grow all together.
If you are thinking out loud to leverage the full potential of these technologies, it is critical that you connect with an expert AI development company with vast industry exposure to think of your business with a unique approach.